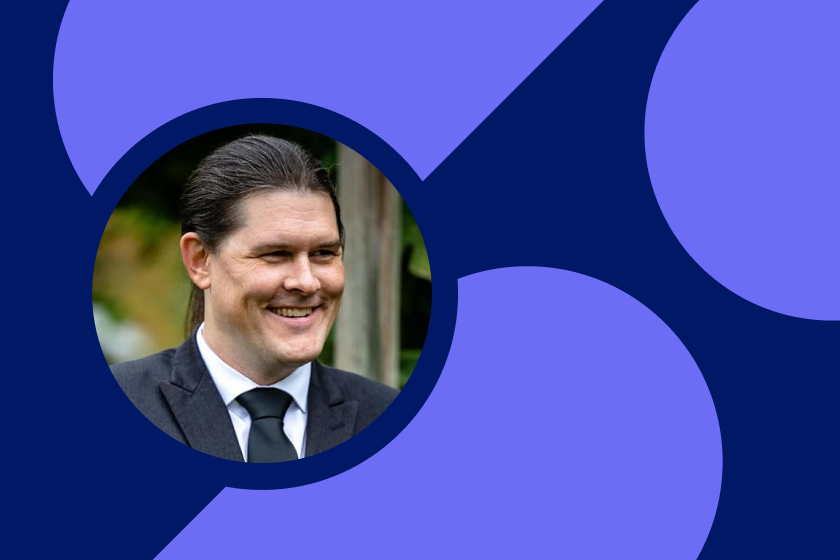
3 ways auto insurers can curb distracted driving in 2024
As technology becomes more addictive, solving for distracted driving becomes more of a challenge. How can auto insurers help measure and manage this behavio…
Read article
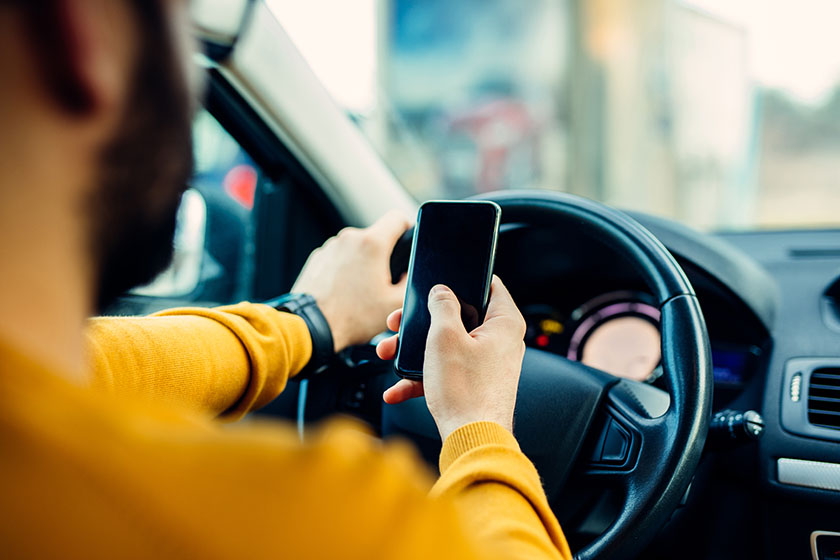
Arity at AIR 2024: Latest driving trends’ impact on auto insurance
Five key takeaways from Arity President Gary Hallgren’s session at Auto Insurance Report (AIR) National Conference.
Read article
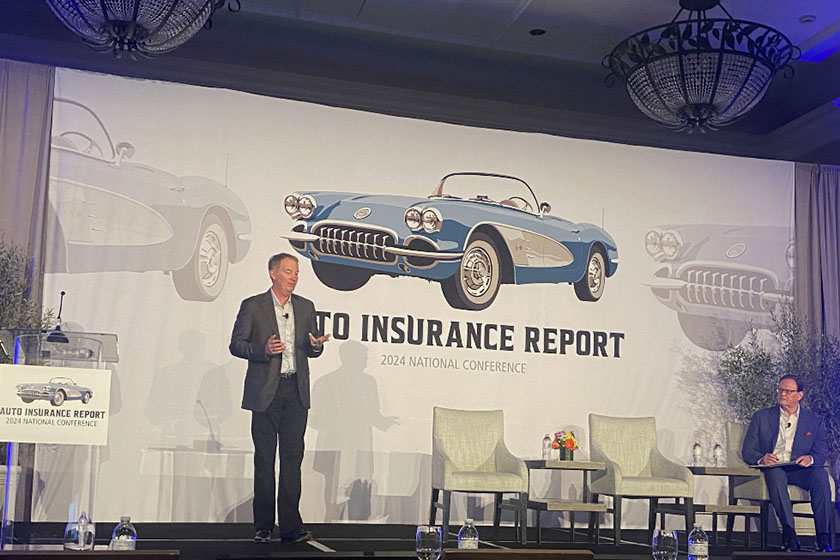
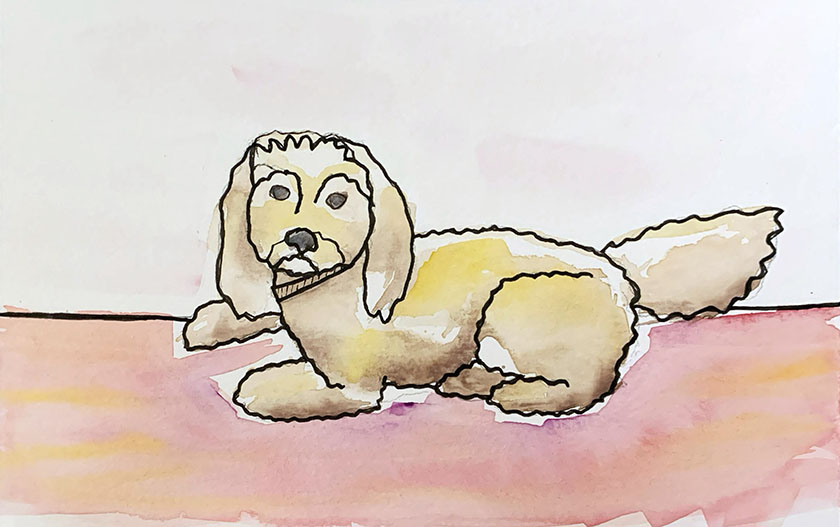
Arity + Clear Channel Outdoor at POSSIBLE: New data, new media for marketers
Director of Product Marketing Jennifer Gold shares three key highlights from her masterclass at POSSIBLE 2024.
Read article
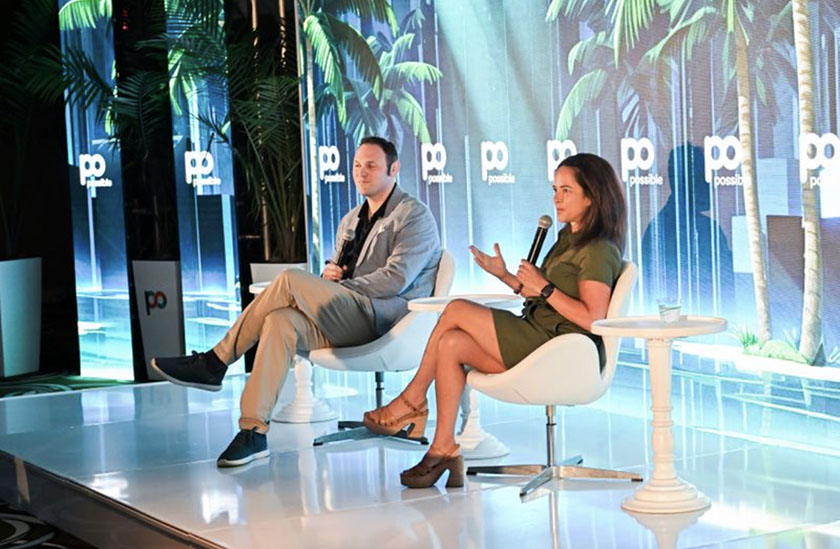